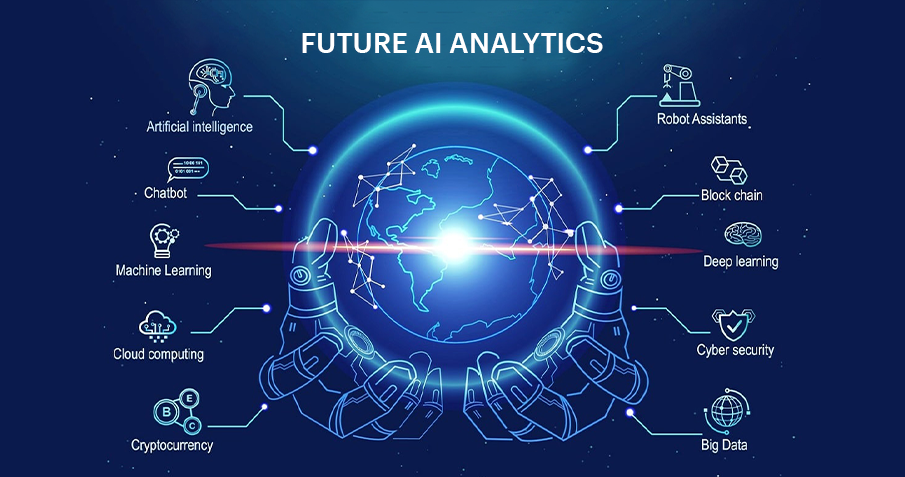
In the future, data analysis is poised to undergo significant transformations and advancements. Key trends shaping the landscape include the integration of artificial intelligence (AI) and machine learning (ML) techniques, enabling automated data preparation, real-time analytics, and enhanced data visualization. With the exponential growth of big data and the Internet of Things (IoT), organizations will need scalable storage solutions and advanced processing frameworks to extract valuable insights. Other important considerations include explainable AI, ensuring privacy, and promoting the democratization of data analysis, empowering users to derive insights and make informed decisions based on data-driven evidence.
The future of data analysis is poised for significant advancements and transformative possibilities.
Several key trends are expected to shape the landscape of data analysis:
- Artificial Intelligence (AI) and Machine Learning (ML): AI and ML techniques are revolutionizing data analysis. These algorithms can process vast amounts of data, identify patterns, make predictions, and generate valuable insights. ML models are becoming increasingly sophisticated, enabling advanced analytics tasks such as anomaly detection, recommendation systems, and predictive modeling.
- Big Data and Internet of Things (IoT): The proliferation of connected devices and IoT is generating massive volumes of data. Future data analysis will need to address the challenges associated with big data, including its volume, velocity, variety, and veracity. Advanced data processing techniques, distributed computing frameworks, and scalable storage solutions will be essential in extracting meaningful insights from big data.
- Real-time Analytics: The demand for real-time analytics is growing with the availability of real-time data streams. Organizations seek immediate insights to take prompt actions based on up-to-date information. Real-time analytics tools and technologies, such as stream processing frameworks, event-driven architectures, and in-memory computing, will play a pivotal role in analyzing data as it arrives.
- Automated Data Preparation: Data preparation tasks, such as cleaning, integration, and transformation, often consume considerable time and effort in data analysis. The future will witness increased automation of these tasks, leveraging AI-powered tools to streamline and expedite data preparation. This will enable analysts to focus more on extracting insights.
- Explainable AI: As AI and ML algorithms become more complex, there is a growing need for explainability and interpretability. Understanding the reasoning behind AI models’ conclusions is crucial for establishing trust, ensuring fairness, and complying with regulatory requirements. Future data analysis methods will incorporate techniques that provide explanations and justifications for AI-driven insights.
- Data Visualization and Storytelling: Effective data visualization techniques will remain crucial in the future of data analysis. As data volumes increase, presenting insights in a visually compelling and understandable manner becomes even more important. Advanced visualization tools, interactive dashboards, and immersive experiences, such as virtual reality (VR) and augmented reality (AR), will enhance data storytelling and enable users to explore data more engagingly.
- Privacy and Security: Growing concerns around data privacy and security necessitate robust protection of sensitive information. Future data analysis will prioritize implementing strong security measures, privacy-enhancing technologies, and compliance with data regulations. Ethical and responsible data analysis practices will be integral to address privacy and security concerns.
- Democratization of Data Analysis: The future of data analysis aims to make it accessible to a broader range of users. Self-service analytics tools, intuitive interfaces, and natural language processing capabilities will empower non-technical users to derive insights from data without heavy reliance on data scientists or analysts. This democratization of data analysis will foster greater data-driven decision-making across organizations.
These trends indicate that the future of data analysis will witness increased automation, advanced analytics techniques, real-time capabilities, and user-friendly tools. As technology continues to evolve, data analysis will become more powerful, enabling organizations to gain deeper insights and make informed decisions based on data-driven evidence.
What is AI Analysis?
AI analysis, also referred to as artificial intelligence analysis, is the process of utilizing artificial intelligence techniques and algorithms to analyze and interpret data. It involves harnessing machine learning, natural language processing (NLP), deep learning, and other AI technologies to extract insights, patterns, and relationships from extensive and intricate datasets.
AI analysis surpasses traditional data analysis methods by empowering machines to autonomously comprehend and process data, generate predictions, and derive meaningful conclusions. It is capable of discovering concealed patterns, identifying anomalies, spotting trends, and offering predictive or prescriptive insights.
The application of AI analysis spans across various domains, including finance, healthcare, marketing, cybersecurity, and more. It encompasses training AI models using historical data and subsequently employing those models to analyze new data, enabling organizations to make data-driven decisions, optimize processes, enhance customer experiences, and identify potential opportunities or risks.
Advancements in AI analysis have given rise to automated data preparation, sophisticated analytics techniques, real-time analysis capabilities, natural language interfaces, and interactive data visualization tools, among others. These technologies facilitate the extraction of valuable insights from data at a faster pace, with increased accuracy, and on a scale that would be arduous for humans to achieve alone.
In summary, AI analysis represents a potent approach to unlocking the inherent potential of data. By providing organizations with deeper insights, improved decision-making capabilities, and a competitive edge in the data-centric landscape, AI analysis holds tremendous promise for the future.
How is AI used in analytics?
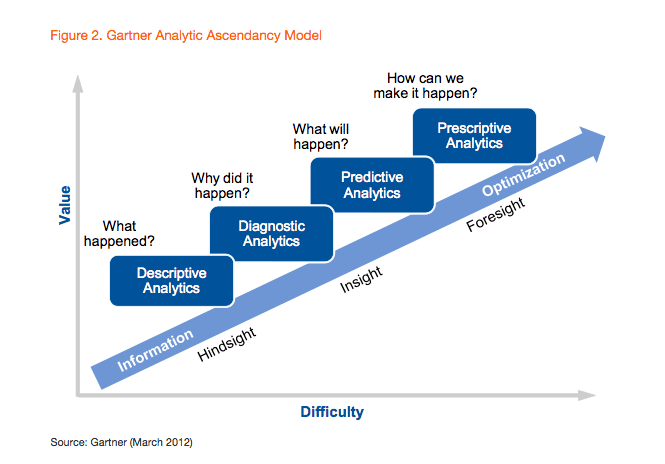
AI is extensively used in analytics to enhance and automate various facets of the data analysis process. Here are some ways in which AI is applied in analytics:
- Data Preparation: AI automates data cleaning, integration, and transformation tasks, reducing the time and effort required. By employing AI algorithms, organizations can handle missing data, outliers, and inconsistencies effectively.
- Pattern Recognition: AI, particularly machine learning, excels at identifying patterns, trends, and correlations within vast and complex datasets. By analyzing historical data, AI models can detect significant relationships and leverage them to generate insights or make predictions.
- Anomaly Detection: AI algorithms are adept at identifying anomalies or outliers in datasets. This capability is valuable in fraud detection, cybersecurity, and quality control, as AI can automatically flag unusual or suspicious patterns for further investigation.
- Natural Language Processing (NLP): NLP empowers AI systems to understand and analyze human language. By leveraging NLP techniques, AI can extract information, sentiment, or intent from text data, enabling applications such as sentiment analysis, text categorization, and chatbot interactions.
- Predictive Analytics: AI enables predictive modeling by utilizing historical data to build models that can forecast future outcomes or behaviors. Predictive analytics finds applications in demand forecasting, predictive maintenance, customer churn prediction, and other similar areas.
- Recommendation Systems: AI-powered recommendation systems analyze user behavior and preferences to provide personalized recommendations. These systems are widely employed in e-commerce, streaming services, and content platforms to suggest products, movies, or articles based on individual preferences.
- Natural Language Interfaces: AI-driven analytics tools often incorporate natural language interfaces, allowing users to interact with data using spoken or written language. This accessibility empowers non-technical users to ask questions, explore data, and receive insights using natural language queries.
- Automated Insights: AI-based analytics platforms can automatically generate insights, trends, and recommendations from data. These systems identify patterns, anomalies, and other noteworthy findings, delivering actionable insights and reducing manual effort in the analysis process.
By leveraging AI in analytics, organizations can automate data preparation, uncover valuable insights, detect anomalies, enable predictive modeling, facilitate natural language interactions, and provide automated insights. This integration enhances efficiency, accuracy, and the ability to derive meaningful insights from data.
What is Data Analysis?
Data analysis is the systematic process of examining, cleaning, transforming, and interpreting data to extract meaningful insights and inform decision-making. It involves several key steps:
- Data Collection: Gathering relevant data from various sources, such as databases, surveys, or sensors.
- Data Cleaning: Ensuring data quality by addressing issues like missing values, outliers, and inconsistencies.
- Data Transformation: Preparing the data for analysis by organizing, aggregating, or reformatting it as needed.
- Exploratory Data Analysis (EDA): Examining the data through statistical summaries, visualizations, and other techniques to understand its characteristics, identify patterns, and uncover initial insights.
- Statistical Analysis: Applying statistical methods to quantify relationships, test hypotheses, and draw inferences from the data. This includes techniques like regression analysis, hypothesis testing, or clustering.
- Data Visualization: Representing data visually through charts, graphs, or interactive dashboards to enhance understanding and communication of insights.
- Interpretation and Decision-making: Drawing conclusions from the analysis, interpreting findings, and using data insights to support informed decision-making.
Data analysis plays a vital role across various fields, including business, finance, healthcare, social sciences, and more. It helps organizations and individuals uncover trends, identify opportunities, solve problems, and optimize performance.
As technology advances, data analysis is also incorporating advanced techniques like machine learning, artificial intelligence, and predictive modeling. These approaches enable more sophisticated analysis, deeper insights, and the ability to make accurate predictions based on complex datasets.
In summary, data analysis is a structured and iterative process that transforms raw data into valuable insights, guiding decision-making and driving improvements across diverse domains.
Top 10 Best AI Tools For Data Analysis
- Python: Python is a versatile programming language known for its rich ecosystem of libraries and frameworks that are widely used in data analysis. It offers popular packages such as NumPy, pandas, scikit-learn, and TensorFlow, making it a preferred choice for data scientists.
- R: R is a programming language designed for statistical computing and data analysis. It provides a comprehensive collection of packages and libraries that enable various statistical analyses, data visualization, and modeling, making it a popular tool among statisticians and data scientists.
- TensorFlow: Developed by Google, TensorFlow is an open-source deep learning library widely used for tasks such as image recognition, natural language processing, and time series analysis. It provides a flexible framework for building and training deep neural networks.
- PyTorch: PyTorch is another popular open-source deep learning library known for its dynamic computational graph and user-friendly interface. It allows for easy prototyping of deep learning models and offers extensive support for tasks like computer vision and natural language processing.
- Scikit-learn: scikit-learn is a Python library that provides a wide range of machine learning algorithms and tools for classification, regression, clustering, and more. It offers user-friendly APIs and functionality for data preprocessing, model evaluation, and feature selection.
- Tableau: Tableau is a powerful data visualization tool that allows users to create interactive dashboards and visualizations. It offers AI-driven features for data exploration, pattern discovery, and storytelling, making it a popular choice for data analysts and business users.
- KNIME: KNIME is an open-source platform that provides a visual workflow interface for data analysis and machine learning. It offers a broad set of pre-built nodes for data preprocessing, modeling, and evaluation, facilitating rapid development and deployment of data analysis workflows.
- RapidMiner: RapidMiner is an end-to-end data science platform that covers the entire data analysis process. It offers a visual interface for building and deploying machine learning models, along with automated feature selection, hyperparameter optimization, and model evaluation capabilities.
- H2O.ai: H2O.ai provides an open-source machine learning platform with distributed computing capabilities. It offers a range of algorithms for regression, classification, clustering, and anomaly detection, and integrates well with popular programming languages like Python and R.
- IBM Watson: IBM Watson is a cloud-based AI platform that offers a suite of tools and services for data analysis, natural language processing, image recognition, and more. It provides AI-powered capabilities for data exploration, predictive modeling, and cognitive insights.
These AI tools serve different purposes and cater to various needs in data analysis. The choice of tool depends on factors such as specific requirements, technical expertise, available resources, and the complexity of the analysis tasks at hand.
How To Use AI for Data Analysis?
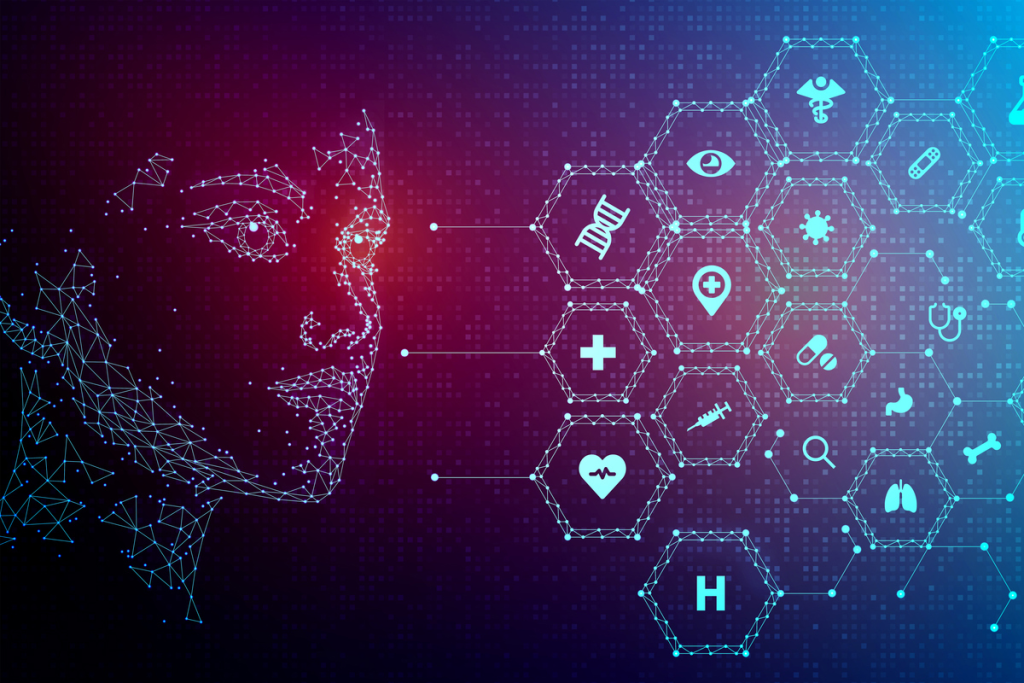
To utilize AI for data analysis, follow these steps:
- Define Objectives: Clearly outline the goals and questions you aim to address through data analysis. Identify the insights or predictions you intend to derive from the data.
- Data Preparation: Collect and preprocess the data for analysis. This involves handling missing values, outliers, and data inconsistencies. Transform the data into a suitable format for AI analysis, such as numerical or categorical variables.
- Choose AI Techniques: Select appropriate AI techniques based on your analysis objectives. This may involve utilizing machine learning algorithms, natural language processing (NLP), deep learning models, or other AI methods.
- Model Training: Train AI models using the prepared data. For supervised learning tasks, ensure the data is appropriately labeled with the desired outcomes or target variables. Reserve a portion of the data for model evaluation.
- Model Evaluation: Assess the performance of the trained AI models using relevant evaluation metrics. This helps gauge how well the models are performing and whether they align with your analysis objectives.
- Generate Insights: Apply the trained AI models to the data to extract insights, predictions, or recommendations. This could involve making predictions on new, unseen data or uncovering patterns and relationships within the existing dataset.
- Visualize and Communicate Results: Utilize data visualization techniques to present the obtained insights and findings in a clear and understandable manner. Visualizations facilitate effective communication with stakeholders and aid in decision-making.
- Iterate and Refine: Analyze the results and iterate as necessary. If the insights generated do not meet the desired objectives, refine the AI models, adjust parameters, or explore alternative approaches to enhance the analysis outcomes.
- Incorporate Human Expertise: While AI algorithms offer powerful capabilities, it is crucial to leverage human expertise for contextual understanding, result interpretation, and domain knowledge incorporation. Combining AI with human insights helps derive comprehensive and meaningful conclusions.
- Ensure Ethical Considerations: Adhere to ethical guidelines in data analysis by respecting privacy, addressing biases, and complying with relevant regulations. Consider the ethical implications of data analysis and strive for transparency and fairness in decision-making.
Remember that AI-based data analysis is an iterative process. As insights are gained, you can refine your approach, update models, or explore additional data sources to enhance the accuracy and relevance of your analysis.
Thanks,
Leave a Reply
You must be logged in to post a comment.